Machine Learning Playbooks: Scale Your Entrepreneurial Venture
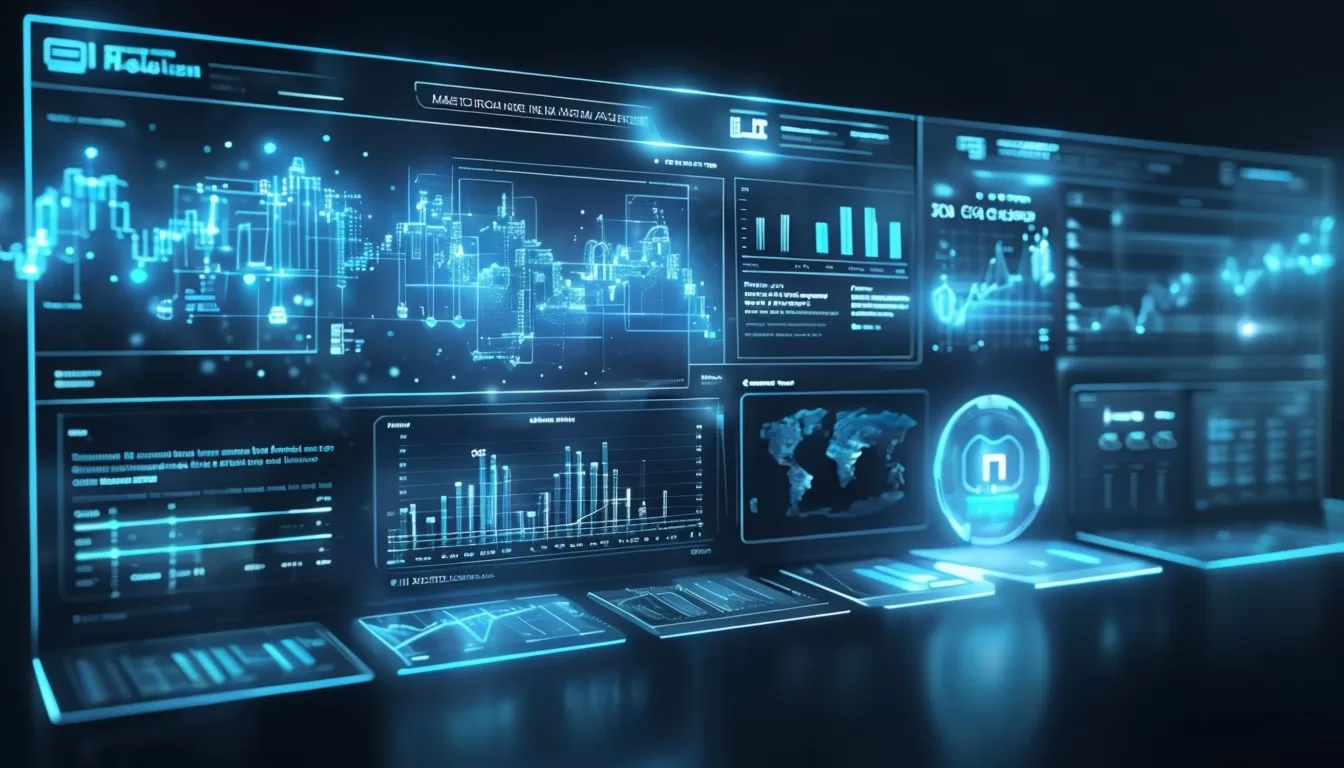
ML Playbooks for Entrepreneurs: Scale Your Business with AI
Are you an entrepreneur feeling overwhelmed by the possibilities of artificial intelligence? Do you know AI can transform your business but struggle to understand where to start? You’re not alone. Many entrepreneurs recognize the potential of machine learning but lack the practical guidance to implement it effectively. The good news is that machine learning playbooks can provide that much-needed direction.
This comprehensive guide provides actionable machine learning playbooks designed specifically for entrepreneurs. We’ll explore practical ML frameworks for entrepreneurs, delve into AI automation blueprints to streamline your operations, and uncover ML strategies for scaling your business to new heights. Forget abstract theory – this is about real-world application.
By the end of this article, you’ll understand:
- The core principles of applying machine learning in an entrepreneurial context.
- How to identify key areas in your business where ML can make a significant impact.
- Step-by-step playbooks for common business challenges, from marketing to operations.
- Practical examples and case studies of successful ML implementations.
- The top AI tools and resources available to entrepreneurs.
- How to build a data-driven culture within your organization.
- Strategies for measuring the ROI of your ML initiatives.
Let’s dive in and unlock the power of machine learning for your entrepreneurial journey.
1. Understanding the Power of Machine Learning Playbooks for Business Growth
Machine learning isn’t just a buzzword; it’s a powerful tool that can revolutionize how you run your business. Machine learning playbooks are essentially structured, repeatable processes that leverage ML algorithms to solve specific business problems. Think of them as strategic blueprints for leveraging AI.
Why are playbooks so important? Because they offer a clear path from problem to solution, reducing the risk and uncertainty associated with implementing AI. They provide a framework for experimentation, learning, and continuous improvement. Without a structured approach, you risk wasting time and resources on projects that don’t deliver tangible results.
Moreover, these playbooks democratize AI, making it accessible to entrepreneurs without requiring a team of data scientists. They allow you to leverage pre-built models, readily available data, and proven methodologies to achieve your business goals. In essence, ML frameworks for entrepreneurs provide a competitive edge in today’s data-driven landscape.
2. Identifying Key Areas for ML Implementation: Where Can AI Make the Biggest Impact?
Before diving into specific playbooks, it’s crucial to identify the areas in your business where machine learning can have the most significant impact. Consider these common pain points and opportunities:
- Marketing: Personalized marketing campaigns, lead scoring, customer segmentation, churn prediction.
- Sales: Sales forecasting, lead prioritization, automated sales workflows, product recommendations.
- Operations: Predictive maintenance, supply chain optimization, fraud detection, process automation.
- Customer Service: Chatbots, sentiment analysis, personalized support experiences, automated ticket routing.
- Product Development: Feature prioritization, market analysis, product personalization, A/B testing optimization.
To identify the best opportunities, ask yourself:
- What are the most time-consuming and repetitive tasks in my business?
- Where am I losing customers or revenue?
- What data do I already have that could be used to improve decision-making?
- What are my competitors doing with AI?
By answering these questions, you can prioritize the areas where AI automation blueprints will deliver the greatest return on investment.
3. Machine Learning Playbook 1: Personalized Marketing Campaigns for Increased Conversions
Personalization is key to effective marketing in today’s crowded digital landscape. This playbook leverages machine learning to deliver targeted messages that resonate with individual customers.
- Step 1: Data Collection and Preparation: Gather data from various sources, including website analytics, CRM, email marketing platforms, and social media. Clean and preprocess the data to ensure accuracy and consistency.
- Step 2: Customer Segmentation: Use clustering algorithms (e.g., K-Means) to segment your customer base based on demographics, behavior, purchase history, and interests.
- Step 3: Content Personalization: Develop personalized content for each segment, including email subject lines, website banners, product recommendations, and ad copy.
- Step 4: Channel Optimization: Use A/B testing and reinforcement learning to optimize the delivery channels for each segment, ensuring that messages are delivered at the right time and in the right format.
- Step 5: Performance Monitoring and Iteration: Track key metrics such as click-through rates, conversion rates, and customer lifetime value. Continuously refine your segmentation and personalization strategies based on performance data.
Example: An e-commerce company could use this playbook to personalize product recommendations on its website, increasing sales by 15%. They could also personalize email marketing campaigns based on past purchases and browsing history, leading to higher open rates and click-through rates.
This playbook is an effective ML strategy for scaling your marketing efforts, allowing you to reach a larger audience with targeted messages that drive conversions.
4. Machine Learning Playbook 2: Predictive Sales Forecasting for Better Resource Allocation
Accurate sales forecasting is essential for effective resource allocation and strategic planning. This playbook uses machine learning to predict future sales based on historical data and market trends.
- Step 1: Data Collection and Preparation: Gather historical sales data, marketing data, economic indicators, and competitor data. Clean and preprocess the data, paying attention to seasonality and trends.
- Step 2: Feature Engineering: Identify relevant features that may influence sales, such as marketing spend, seasonality, economic indicators, and competitor activity.
- Step 3: Model Selection and Training: Choose a suitable regression model, such as linear regression, polynomial regression, or time series models like ARIMA or Prophet. Train the model on historical data.
- Step 4: Model Evaluation and Tuning: Evaluate the model’s performance using metrics such as Mean Absolute Error (MAE) and Root Mean Squared Error (RMSE). Tune the model parameters to improve accuracy.
- Step 5: Sales Forecasting and Deployment: Use the trained model to predict future sales. Deploy the model to a sales dashboard or reporting system for easy access.
Example: A SaaS company could use this playbook to predict future subscription revenue, allowing them to allocate resources more effectively and plan for future growth. They could also use the forecast to identify potential sales shortfalls and take corrective action.
This playbook provides valuable insights for ML strategies for scaling your sales operations and ensuring sustainable growth.
5. Machine Learning Playbook 3: Optimizing Customer Service with AI-Powered Chatbots
Customer service is a critical touchpoint for building brand loyalty and driving revenue. This playbook uses AI-powered chatbots to provide instant and personalized support to customers.
- Step 1: Define Chatbot Goals and Use Cases: Identify the specific customer service tasks that the chatbot will handle, such as answering FAQs, resolving common issues, or providing product recommendations.
- Step 2: Data Collection and Training: Gather customer service transcripts, FAQs, and product documentation. Train the chatbot using natural language processing (NLP) techniques to understand and respond to customer inquiries.
- Step 3: Chatbot Design and Development: Design the chatbot’s conversational flow and user interface. Integrate the chatbot with your existing customer service systems.
- Step 4: Testing and Deployment: Test the chatbot thoroughly to ensure that it can handle a wide range of customer inquiries. Deploy the chatbot to your website, mobile app, or social media channels.
- Step 5: Monitoring and Improvement: Monitor the chatbot’s performance and gather customer feedback. Continuously improve the chatbot’s accuracy and effectiveness based on user interactions.
Example: A retail company could use this playbook to deploy a chatbot on its website to answer customer questions about product availability, shipping costs, and return policies. The chatbot could also provide personalized product recommendations based on customer browsing history.
This is an important AI automation blueprint to improve customer satisfaction and reduce operational costs.
6. Top AI Tools for Entrepreneurs: Empowering Your Business with Cutting-Edge Technology
To effectively implement these machine learning playbooks, entrepreneurs need access to the right tools. Here are some of the top AI tools available:
- Google Cloud AI Platform: A comprehensive platform for building, training, and deploying machine learning models. Offers a wide range of pre-trained models and custom model development tools.
- Amazon SageMaker: A fully managed machine learning service that enables developers and data scientists to quickly and easily build, train, and deploy machine learning models at any scale.
- Microsoft Azure Machine Learning: A cloud-based platform for building, deploying, and managing machine learning models. Offers a wide range of tools and services for data preparation, model training, and model deployment.
- DataRobot: An automated machine learning platform that enables users to build and deploy machine learning models without writing code.
- H2O.ai: An open-source machine learning platform that provides a wide range of algorithms and tools for data analysis, model building, and model deployment.
- Jasper: An AI-powered content creation tool that can generate high-quality content for marketing, sales, and other business needs.
- Zapier: An automation platform that allows you to connect different apps and services to automate repetitive tasks and workflows.
These tools are essential components of successful ML frameworks for entrepreneurs, allowing you to leverage the power of AI without needing extensive technical expertise.
7. Building a Data-Driven Culture: Fostering Innovation and Informed Decision-Making
Implementing machine learning successfully requires more than just technology; it requires a data-driven culture that values experimentation, learning, and continuous improvement.
- Educate Your Team: Provide training and resources to help your team understand the basics of machine learning and data analysis.
- Empower Data-Driven Decision-Making: Encourage your team to use data to inform their decisions, rather than relying on gut feeling or intuition.
- Promote Experimentation: Create a safe environment for experimentation, where failure is seen as an opportunity to learn and improve.
- Share Data and Insights: Make data and insights readily available to everyone in your organization.
- Celebrate Successes: Recognize and reward employees who use data to achieve positive results.
By fostering a data-driven culture, you can unlock the full potential of machine learning and drive innovation across your organization. This contributes significantly to the effectiveness of your machine learning playbooks.
8. Measuring the ROI of Your ML Initiatives: Proving the Value of AI
It’s crucial to measure the return on investment (ROI) of your machine learning initiatives to justify the cost and demonstrate the value of AI to your stakeholders.
- Define Key Performance Indicators (KPIs): Identify the specific metrics that you will use to measure the success of your ML initiatives, such as increased revenue, reduced costs, or improved customer satisfaction.
- Establish a Baseline: Measure the current performance of your business before implementing any ML initiatives.
- Track Performance Over Time: Monitor the performance of your business after implementing ML initiatives and compare it to the baseline.
- Calculate ROI: Calculate the ROI of your ML initiatives by dividing the net profit by the cost of investment.
- Communicate Results: Communicate the results of your ROI analysis to your stakeholders, highlighting the benefits of AI and the potential for future growth.
By measuring the ROI of your ML initiatives, you can demonstrate the value of AI and secure the resources needed to continue investing in this transformative technology. This validation is critical for continued support and expansion of your ML strategies for scaling.
9. Overcoming Common Challenges in ML Implementation: Addressing Potential Roadblocks
While the potential of machine learning is immense, there are also challenges to be aware of. Here are some common roadblocks and how to overcome them:
- Lack of Data: Ensure you have sufficient, high-quality data. Consider data augmentation techniques or partnering with external data providers.
- Lack of Expertise: Invest in training your team or hire data scientists with the necessary skills. Consider using automated machine learning platforms to simplify the process.
- Integration Challenges: Ensure that your ML models can be seamlessly integrated with your existing systems. Use APIs and cloud-based platforms to facilitate integration.
- Model Bias: Be aware of potential biases in your data and models. Use fairness-aware machine learning techniques to mitigate bias and ensure equitable outcomes.
- Ethical Considerations: Consider the ethical implications of your ML applications. Ensure that your models are used responsibly and in compliance with relevant regulations.
By anticipating and addressing these challenges, you can increase the likelihood of success with your machine learning initiatives. Having mitigation strategies planned is crucial for effective implementation of your machine learning playbooks.
10. Future Trends in Machine Learning for Entrepreneurs: Staying Ahead of the Curve
The field of machine learning is constantly evolving, with new algorithms, tools, and applications emerging all the time. Here are some future trends that entrepreneurs should be aware of:
- Edge Computing: Deploying ML models on edge devices, such as smartphones and IoT devices, to enable real-time processing and reduced latency.
- Federated Learning: Training ML models on decentralized data sources without sharing the data itself, enabling privacy-preserving machine learning.
- Explainable AI (XAI): Developing ML models that are transparent and interpretable, allowing users to understand how the models make decisions.
- Generative AI: Using AI to generate new content, such as images, text, and code, enabling creative applications and personalized experiences.
- Reinforcement Learning: Training ML models to make optimal decisions in dynamic environments, enabling applications such as robotics and autonomous systems.
By staying ahead of these trends, entrepreneurs can position themselves to leverage the latest advancements in machine learning and gain a competitive edge. Continuously learning and adapting is crucial for maximizing the benefits of ML frameworks for entrepreneurs.
Frequently Asked Questions
1. What exactly is a machine learning playbook, and how does it differ from a standard business plan?
A machine learning playbook is a structured, repeatable process that uses machine learning algorithms to solve specific business problems. Unlike a standard business plan, which outlines overall business strategy, a playbook focuses on leveraging AI to address targeted challenges. It includes specific steps for data collection, model selection, training, deployment, and evaluation. Think of it as a tactical guide to applying AI for a specific purpose, offering a more granular and actionable approach than a broad business strategy. It outlines precisely how machine learning will be implemented to achieve a defined objective.
2. How much technical expertise is required to implement these machine learning playbooks?
While some technical knowledge is helpful, it’s not always necessary to be a data scientist to implement these playbooks. Many AI tools and platforms offer user-friendly interfaces and automated features that simplify the process. Focus on understanding the business problem you’re trying to solve and leveraging available resources to guide your implementation. Consider using no-code or low-code AI platforms, which allow you to build and deploy ML models without writing code. You may need to collaborate with technical experts for more complex projects, but starting with simpler playbooks can build your confidence and understanding.
3. What are the initial data requirements for starting with these machine learning playbooks?
The data requirements vary depending on the specific playbook you’re implementing. However, you generally need historical data related to the problem you’re trying to solve. For example, for personalized marketing, you’ll need customer data, website analytics, and email marketing data. Ensure the data is clean, accurate, and relevant. Start small with a manageable dataset and gradually expand as you gain experience. If you lack sufficient data, consider data augmentation techniques or partnering with external data providers.
4. How can I ensure the data I’m using for machine learning is unbiased and ethical?
Ensuring data is unbiased and ethical is crucial for responsible AI implementation. Review your data sources for potential biases and address them through data preprocessing techniques. Use fairness-aware machine learning algorithms that are designed to mitigate bias. Regularly audit your models for bias and monitor their impact on different demographic groups. Establish clear ethical guidelines for data collection and usage within your organization. Transparency and accountability are essential for building trust in your AI systems.
5. How do I choose the right machine learning tools and platforms for my business?
Choosing the right tools depends on your specific needs, budget, and technical expertise. Consider factors such as ease of use, scalability, integration capabilities, and pricing. Start with free trials or open-source options to experiment with different platforms. Read reviews and compare features before making a decision. If you’re new to machine learning, opt for platforms with automated features and user-friendly interfaces. As your expertise grows, you can explore more advanced tools and platforms.
6. How often should I update and retrain my machine learning models?
The frequency of updating and retraining your models depends on the stability of your data and the environment in which they operate. In dynamic environments, where data patterns change rapidly, you may need to retrain your models more frequently, such as weekly or monthly. In more stable environments, you can retrain them less often, such as quarterly or annually. Monitor your model’s performance regularly and retrain it whenever you notice a significant drop in accuracy or performance. Implement automated retraining pipelines to streamline the process.
7. What are some common mistakes entrepreneurs make when implementing machine learning?
Common mistakes include starting with overly complex projects, neglecting data quality, failing to define clear goals, and lacking a data-driven culture. Avoid these mistakes by starting small, focusing on data quality, defining clear objectives, and fostering a data-driven mindset within your organization. Don’t underestimate the importance of communication and collaboration between technical and business teams. Be prepared to iterate and adapt your approach based on results and feedback.
8. How can I convince my team and stakeholders to embrace machine learning initiatives?
Convincing your team and stakeholders requires clear communication, demonstrating value, and addressing concerns. Explain the benefits of machine learning in terms of increased revenue, reduced costs, and improved customer satisfaction. Start with pilot projects that deliver tangible results and showcase the potential of AI. Address concerns about job displacement and emphasize the role of AI in augmenting human capabilities. Provide training and resources to help your team understand and embrace machine learning.
9. What are the legal and regulatory considerations when using machine learning?
Legal and regulatory considerations include data privacy, data security, and algorithmic bias. Comply with relevant regulations such as GDPR and CCPA when collecting and using personal data. Implement robust security measures to protect your data from breaches. Ensure that your models are fair and unbiased to avoid discriminatory outcomes. Consult with legal experts to ensure that your AI applications comply with all applicable laws and regulations.
10. What resources are available to help me learn more about machine learning for entrepreneurs?
Numerous resources are available, including online courses, books, articles, and communities. Consider platforms like Coursera, edX, and Udacity for structured learning. Read books on machine learning and AI for business. Join online communities and forums to connect with other entrepreneurs and experts. Attend industry events and conferences to stay up-to-date on the latest trends and technologies. Leverage resources from AI tool vendors and cloud providers.
Key Takeaways
- Machine learning playbooks provide structured, repeatable processes for leveraging AI to solve specific business problems.
- Identifying key areas for ML implementation, such as marketing, sales, and customer service, is crucial for maximizing ROI.
- Personalized marketing campaigns, predictive sales forecasting, and AI-powered chatbots are powerful applications of machine learning for entrepreneurs.
- Top AI tools such as Google Cloud AI Platform, Amazon SageMaker, and DataRobot empower entrepreneurs to build and deploy ML models.
- Building a data-driven culture that values experimentation, learning, and continuous improvement is essential for successful ML implementation.
- Measuring the ROI of your ML initiatives is crucial for justifying the cost and demonstrating the value of AI to stakeholders.
- Addressing common challenges such as lack of data, lack of expertise, and integration challenges is essential for overcoming potential roadblocks.
- Staying ahead of future trends in machine learning, such as edge computing and federated learning, will provide a competitive edge.
- Ethical considerations, including data privacy and algorithmic bias, must be addressed to ensure responsible AI implementation.
- Continuously learning and adapting is crucial for maximizing the benefits of machine learning for your entrepreneurial journey.